IMPRS Seminar - Tandem Talk with Kirandeep Kour and Prof. Dr. Peter Benner
Low-rank Tensor Decompositions in Kernel-based Machine Learning
During our IMPRS Seminar on January 13, 2023, a tandem talk was held by Prof. Dr. Peter Benner and Kirandeep Kour. Tandem talks are part of the IMPRS program and an excellent opportunity for researchers to exchange current findings and challenges. This Seminar took place in a hybrid format, with the possibility to join the talk in person at the Max-Planck-Institut or online. Kirandeep Kour talked about the research of her PhD topic, while Prof. Dr. Peter Benner gave an introduction to some Methods in Machine Learning to give a basis for understanding the research topic "Low-rank Tensor Decompositions in Kernel-based Machine Learning".
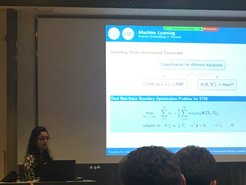
After the introductory session held by Prof. Dr. Peter Benner, Kirandeep Kour followed with a detailed description of the work with some numerical experiments and results.
The talk dealed with the problem, that in several scientific fields like neuroscience, medical science and signal processing, enormous amounts of data are being generated. Generally, these data depend on various parameters and thus can be interpreted as multidimensional data (tensor) and incorporate structure in the multidimensionality. Due to this dimensionality, it usually requires a high computational cost when working with tensor datasets. The Low-rank tensor decompositions (LRTD) reduce the afore mentioned issue and aim at providing a natural and compact structure-preserving representation. In the doctoral project of Kirandeep Kour, they have worked on the development of LRTD algorithms for binary classification with small sample-sized datasets like functional Magnetic Resonance Imaging (fMRI), CT scans, and Hyper-spectral Images. The kernel computation (feature extraction technique) in the Kernel-based method such as Support Tensor Machine (extended version of Support Vector Machine for tensor data) benefits from newly established LRTD methods. The benefits include achieving a robust, computationally efficient machine learning model along with state-of-the-art classification accuracy.
We thank everyone who participated, and especially the two speakers.