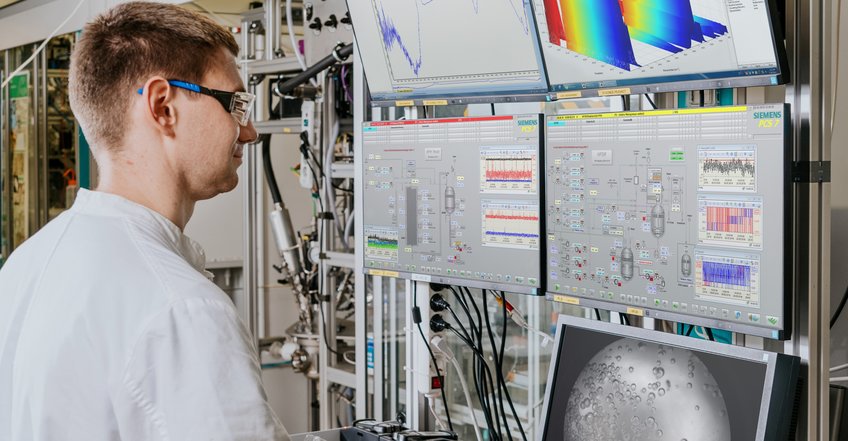
The Process Systems Engineering (PSE) Group
Technical systems of ever-increasing complexity are changing our environment to a dramatic extent. Research on these systems is ultimately triggered by the key question: How can Earth’s resources be utilized in a sustainable manner?
Vision
In recent decades, we have seen continuous progress in increasing the productivity and selectivity of (bio-)chemical and energy conversion processes. Nevertheless, in order to cope with the grand challenges of the future, new breakthroughs in Process Systems Engineering are necessary in order to achieve drastic performance improvements in existing processes, to invent dream process technologies for synthesizing chemicals and transforming energy to the highest level of efficiency, to strongly accelerate the transition from fossil to renewable raw materials, to make process operation much more flexible, to organize a comprehensive circular economy for as many material streams as possible, and - at the same time - to achieve even higher product quality and functionality objectives.
For this purpose, scientifically-founded process systems engineering methodologies that are able to deal with the inherent multi-level structure of chemical production systems need to be developed. Highly efficient process systems can be designed if engineers succeed in considering each hierarchical level involved in a process system simultaneously, i.e. from the molecular level up to the level of an interconnected system of production plants (see Fig.1). A multi-level design workflow must be fed with reliable thermodynamic and kinetic models and be validated and parameterized by use of experimental data at different levels of the process hierarchy. Harvesting experimental data is also indispensable for discriminating between rival models, for example of reaction kinetic networks, and for achieving uncertainty quantification of model predictions. Hence, only by closely combining mathematical modeling and systematically collecting experimental data can an advanced quantitative understanding of complex process systems be accomplished in order to open up new pathways for translating scientific advance¬ments into practical solutions.
Furthermore, natural living systems feature unique properties, which have so far never been observed in the world of technical systems, for example the ability of cells to self-replicate, the self-adaptivity of living cellular communities to large perturbations of environmental conditions, or the high specificity of many enzymes acting as catalysts in metabolic reaction networks. Long-term, it would be fantastic to mimic the functional principles of biological systems in order to understand how they work, and to create new “screwdrivers” for the toolboxes of systems engineering. This might become reality if systems engineering principles could be combined successfully with bottom-up synthetic biology approaches.
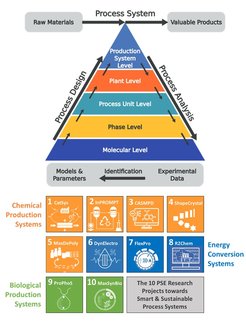
Mission
The above vision forms the background to the PSE group’s long-term research strategy:
- Development of a generalized multiscale process design methodology, the Elementary Process Functions (EPF) methodology [1-4], which systematically considers all available decision variables on the different hierarchical levels of a production system. More recently, it has evolved towards the FluxMax approach [5, 6]. Moreover, we also developed novel concepts and methods for enabling the molecular selection and design of process materials (e.g. solvents, adsorbents), as well as for integrating molecular, material and process design [7-11].
- Computer-aided design of sustainable processes for the production of bulk and platform chemicals (e.g. for Cl2 [12], and aldehydes [13]), chemical conversion of renewable energy (e.g. syngas [14], CH4 [15, 16], CH3OH [6]), and innovative biotechnology (microalgae-based biorefinery [17, 18], synthetic cells [19-21]). We are focusing on processes operated with renewable feedstock (biomass, water, air, recyclates), driven by energy from renewables (sun, wind), equipped with environmentally friendly materials, featuring high flexibility, safety and economical attractiveness.
- Experimental evaluation of promising novel process concepts (derived with the theoretical and computational methods mentioned above) by use of advanced inline/online/offline analytical tools, automatized miniplants, and microfluidic devices. In the last few years, amongst others, our group has constructed and operated several complex miniplants (hydroformylation of alkenes [22], CO2 methanation [23]) and has presented a novel microfluidic chip design for producing cell-like compartments [24, 25].
Selected Publications of the PSE Group
[1] Freund, H., & K. Sundmacher (2008). Towards a methodology for the systematic analysis and design of efficient chemical processes – Part 1: From unit operations to elementary process functions. Chemical Engineering and Processing: Process Intensification 47, 2051-2060.
[2] Freund, H., & K. Sundmacher (2011). Process intensification: 1. Fundamentals and molecular level, 2. Phase level, 3. Process unit level, 4. Plant level, in: Ullmann´s Encyclopedia of Industrial Chemistry, Wiley-VCH, Weinheim.
[3] Peschel, A., Freund, H., & K. Sundmacher (2010). Methodology for the Design of Optimal Chemical Reactors Based on the Concept of Elementary Process Functions. Ind. Eng. Chem. Res., 49 (21), 10535-10548.
[4] Kaiser, N. M., Flassig, R.J., & K. Sundmacher (2018). Reactor-network synthesis via flux profile analysis. Chemical Engineering Journal, 335, 1018-1030.
[5] Liesche, G., Schack, D., & K. Sundmacher (2019). The FluxMax Approach for Simultaneous Process Synthesis and Heat Integration: Production of Hydrogen Cyanide. A.I.Ch.E. Journal, 65 (7), e16554.
[6] Schack, D., Liesche, G., & K. Sundmacher (2020). The FluxMax approach: Simultaneous flux optimization and heat integration by discretization of thermodynamic state space illustrated on methanol synthesis process. Chemical Engineering Science, 215: 115382.
[7] Song, Z., Zhang, C., Qi , Z., Zhou, T., & Sundmacher, K. (2018). Computer-aided design of ionic liquids as solvents for extractive desulfurization. A.I.Ch.E. Journal, 64 (3), 1013–1025.
[8] Zhou, T., Song, Z., & Sundmacher, K. (2019). Big Data Creates New Opportunities for Materials Research: A Review on Methods and Applications of Machine Learning for Materials Design. Engineering, 5 (6), 1017–1026.
[9] Zhou, T., McBride, K., Linke, S., Song, Z., & Sundmacher, K. (2020). Computer-aided solvent selection and design for efficient chemical processes. Current Opinion in Chemical Engineering, 27, 35-44.
[10] Zhang, X., Ding, X., Song, Z., Zhou, T., & Sundmacher, K. (2021). Integrated ionic liquid and rate-based absorption process design for gas separation: global optimization using hybrid models. A.I.Ch.E. Journal, 67 (10), e17340.
[11] Zhang, X., Zhou, T., & Sundmacher, K. (2021). Integrated metal–organic framework and pressure/vacuum swing adsorption process design: Descriptor optimization. A.I.Ch.E. Journal, e17524; doi.org/10.1002/aic.17524.
[12] Bechtel, S., Vidaković-Koch, T., & K. Sundmacher (2018). Novel process for the exergetically efficient recycling of chlorine by gas phase electrolysis of hydrogen chloride. Chemical Engineering Journal, 346, 535–548.
[13] Rätze, K., Jokiel, M., Kaiser, N. M., & K. Sundmacher (2019). Cyclic Operation of a Semi-Batch Reactor for the Hydroformylation of Long-Chain Olefins and Integration in a Continuous Production Process. Chemical Engineering Journal, 377, 120453.
[14] Maggi, A., Wenzel, M., & K. Sundmacher (2020). Mixed-Integer Linear Programming (MILP) Approach for the Synthesis of Efficient Power-to-Syngas Processes. Frontiers in Energy Research, 8, 161.
[15] Zimmermann, R. T., Bremer, J., & K. Sundmacher (2020). Optimal catalyst particle design for flexible fixed-bed CO2 methanation reactors. Chemical Engineering Journal, 387, 123704.
[16] Zimmermann, R. T., Bremer, J., & K. Sundmacher (2022). Load-flexible fixed-bed reactors by multi-period design optimization. Chemical Engineering Journal, 428, 130771.
[17] Fachet, M., Hoeffner, K., Barton, P. I. & K. Sundmacher (2016). Dynamic flux balance modeling to increase the production of high-value compounds in green microalgae. Biotechnology for Biofuels, 9, 165.
[18] König-Mattern, L., Linke, S., Rikho-Struckmann, L. & K. Sundmacher (2021). Computer-aided solvent screening for the fractionation of wet microalgae biomass. Green Chemistry, in press, doi.org/10.1039/D1GC03471E.
[19] Rollié, S., Mangold, M., & K. Sundmacher (2012). Designing biological systems: systems engineering meets synthetic biology. Chemical Engineering Science, 69, 1-29.
[20] Schwille, P., Spatz, J. P., Landfester, K., Bodenschatz, E., Herminghaus, S., Sourjik, V., Erb, T., Bastiaens, P., Lipowsky, R., Hyman, A. A., Dabrock, P., Baret, J.-C., Vidaković-Koch, T., Bieling, P., Dimova, R., Mutschler, H., Robinson, T., Tang, T.-Y. D., Wegner, S., & K. Sundmacher (2018). MaxSynBio: Avenues towards creating cells from the bottom up. Angewandte Chemie International Edition, 57 (41), 13382–13392.
[21] Ivanov, I., Lopez-Castellanos, S., Balasbas, S., Otrin, L., Marusic, N., Vidaković-Koch, T., & K. Sundmacher (2021). Bottom-Up Synthesis of Artificial Cells: Recent Highlights and Future Challenges. Annual Reviews in Chemical and Biomolecular Engineering, 12, 287-308.
[22] Jokiel, M., Kaiser, N. M., Kováts, P., Mansour, M., Zähringer, K., Nigam, K. D. P., & K. Sundmacher (2019). Helically coiled segmented flow tubular reactor for the hydroformylation of long-chain olefins in a thermomorphic multiphase system. Chemical Engineering Journal, 377: 120060.
[23] Bremer, J. (2020). Advanced Operating Strategies for Non-Isothermal Fixed-Bed Reactors, Exemplified for CO2 Methanation. PhD Thesis, Magdeburg, Otto-von-Guericke-Universität.
[24] Weiss, M., Frohnmayer, J. P., Benk, L. T., Haller, B., Janiesch, J.-W., Heitkamp, T., Börsch, M., Lira, R. B., Dimova, R., Lipowsky, R., Bodenschatz, E., Baret, J.-C., Vidaković-Koch, T., Sundmacher, K., Platzman, I., & J. P. Spatz (2018). Sequential bottom-up assembly of mechanically stabilized synthetic cells by microfluidics. Nature Materials, 17, 89–96.
[25] Krafft, D., Lopez-Castellanos, S., Dimova, R., Ivanov, I., & K. Sundmacher (2019). Compartments for Synthetic Cells: Osmotically Assisted Separation of Oil from Double Emulsions in Microfluidic Chip. ChemBioChem: A European Journal of Chemical Biology, 20 (20), 2604–2608.