Sridhar Chellappa successfully defended his PhD thesis!
Research focused on Model Order Reduction and A Posteriori Error Estimation
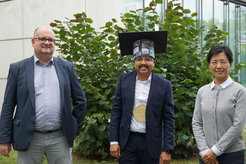
Sridhar Chellappa, one of our IMPRS doctoral researchers, successfully defended his PhD thesis on September 13, 2022, on the topic of “A Posteriori Error Estimation and Adaptivity for Model Order Reduction of Large-Scale Systems”. He joined the Max-Planck Institute in April 2017 and later joined the IMPRS in the 3rd year of his PhD, in May 2019, where he worked in the group of Prof. Benner “Computational Methods in Systems and Control Theory” in the team headed by Dr. Lihong Feng on “Model Order Reduction”.
The use of computer simulations to model and understand physical phenomena has become routine in many areas of science and technology such as process engineering, chemistry, fluid dynamics, etc. As models grow more complicated, the computational cost of these simulations has risen dramatically. While hardware-based solutions such as high-performance clusters or graphic-process units (GPUs) can help cope with this, they carry a high price tag.
Sridhar Chellappa's PhD project focused on model order reduction (MOR), a set of computational methods that enable speeding up simulations while maintaining the accuracy of the reduced model. While several MOR methods already exist, they often carry a large up-front computational cost. In his work, he introduced adaptive methods that substantially reduce the up-front cost of MOR algorithms. He dealt with the adaptivity of model updates and parameter sampling.
The driving force behind the adaptive MOR methods is a posteriori error estimation. Through theoretical analysis, he developed new error estimators for different classes of systems. The developed error estimators are able to certify how accurate the reduced order model is. Using this information, the reduced model can be refined iteratively.
He applied the adaptive MOR methods to different benchmark models such as batch chromatography, fluidized bed crystallizer, and electromagnetic devices. The adaptive MOR methods were able to reduce the up-front computational cost by up to 30%. Moreover, the adaptive sampling of the model parameters leads to reduced models that generalize much better compared to standard approaches.
Being a part of MPI in Magdeburg for the last 5 years has been a fantastic experience, he said. He immensely enjoyed the working atmosphere, support, and resources he got as a PhD researcher. He mentioned that colleagues were always ready to talk and help out with issues both technical and beyond (such as help with settling down, German translations, etc.). He highlighted especially the International Office. When he first came to Germany, he was given all possible help to make himself feel at home in Magdeburg.
The daily atmosphere in the CSC group of Prof. Peter Benner and in the Team Model Order Reduction headed by Dr. Lihong Feng. was very motivating. The mentorship and supervision were consistent and good. He particularly liked the chance to travel early in his PhD to Summer Schools and Conferences. This helped him to get in touch with the larger scientific community and helped forge future collaborations.
Sridhar Chellappa stated that the structured programme of the IMPRS helps define a clear path for PhD students, which he feels is quite important. Another great aspect of IMPRS to him was the possibility to do soft-skills courses. He took part in an Effective Communications workshop and learned a lot about the nuances of good communication. He said, he appreciates the effort taken by the IMPRS team in keeping alive the activities even during the pandemic days.
After defending his thesis, he currently works as a postdoc in the CSC group. He will work on new approaches that combine MOR methods with ideas from the field of Machine learning.
Congratulations on your successful defence and we wish you all the best!