Linking of data-driven and physics-based modeling in electrochemical energy conversion processes
Successful commercialization of electrochemical systems requires an improvement of their performance, reliability, and durability. In order to achieve these goals, many studies have been devoted to the development of diagnostic methodologies for the early detection of material degradation symptoms, identification of the causes of degradation mechanisms, and development of mitigation strategies to extend the lifetime of these devices. The most popular technique used to diagnose different kinds of degradation mechanisms is electrochemical impedance spectroscopy (EIS). However, EIS often fails to separate the contributions of single processes to the overall performance losses, due to the coupling in the frequency spectra of dynamic phenomena with similar time constants. In order to overcome these limitations, different mathematical procedures have been employed to analyze the EIS spectra with the aim to decouple the values of the different resistance losses embedded in them. The most successful among these approaches are the ones based on the determination of the distribution function of relaxation times (DFRT). However, the determination of the DRT function is not a trivial task because the underlying problem is inverse ill-posed. For this reason, this kind of analysis is dependent on initial assumptions based on previous knowledge of the physical behavior of the system, which is not always well established and can lead to wrong conclusions.
The Loewner framework (LF) is a versatile, and easy to implement data driven modeling method that computes reduced-order models in state-space representation through a forward approach directly from frequency response data. It allows to obtain the distribution of the time constants or characteristic frequencies bypassing the problems encountered with the inversion methods used for the determination of the DFRT.
In [1,2], we successfully demonstrated that the application of the LF for the analysis of EIS data of a polymer electrolyte membrane fuel cell (PEMFC) system facilitates the interpretation of the related frequency domain spectra and allows clear identification of the individual polarization processes based on their characteristic frequencies, as shown in Fig.1. Hence, unambiguous fault identification and monitoring of the degradation state of the cell is possible and it can be the ground of an onboard diagnostic tool.
In this project, we are going to establish the connection between physics and machine learning (ML) or deep learning (DL) modeling in electrochemical energy conversion processes through LF-based approach. Also, we will extend the application of this LF-based approach to different electrochemical systems, i.e. batteries, and different types of fuel cells and electrolyzers.
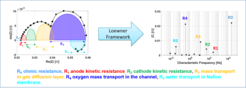
Funding: IMPRS
Collaborations:
Prof. Antoulas, MPI Magdeburg & Rice University Houston, USA
Selected recent publications:
[1] B. Patel, “Application of Loewner framework for data-driven modeling and diagnosis of polymer electrolyte membrane fuel cells”, Master Thesis, Otto-von-Guerike Universität, 2021, https://opendata.uni-halle.de//handle/1981185920/103283, DOI: 10.25673/101328.
[2] Bansidhar Patel, Antonio Sorrentino, Ion Victor Gosea, Athanasios C. Antoulas, Tanja Vidakovic-Koch, “Application of Loewner framework for data-driven modeling and interpretation of impedance spectra of polymer electrolyte membrane fuel cells”, EFCF, 2021.