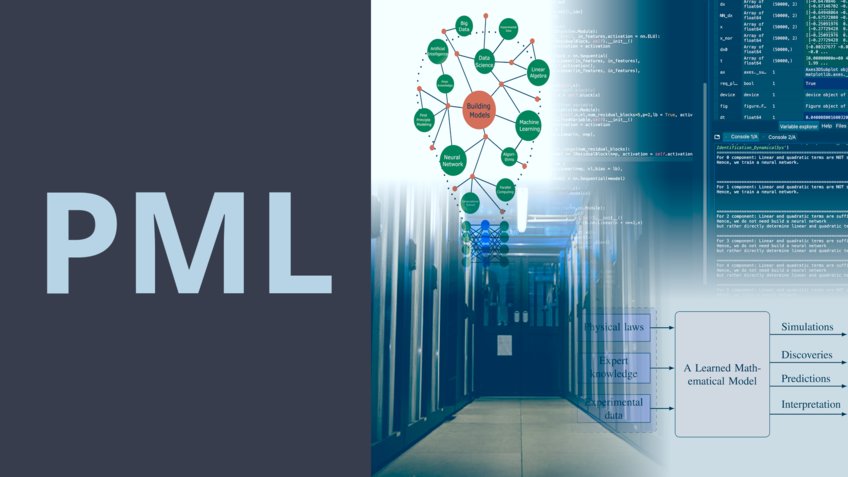
Physics-Enhanced Machine Learning
With the rapid development in measurement technology and computing power, our interest lies in learning models that explain underlying dynamical behaviors and draw meaningful conclusions from the learned model. The PML team is particularly dedicated to developing innovative machine learning methodologies by incorporating fundamental principles and empirical knowledge of the process. Moreover, we focus on inferring low-dimensional models using machine learning tools, thus reducing computational cost of high-fidelity models.
Projects
Feature Publications
56, pp. 28 - 51 (2022)
Operator Inference and Physics-Informed Learning of Low-Dimensional Models for Incompressible Flows. Electronic Transactions on Numerical Analysis: Special Issue SciML
372, 113433, 17 pages (2020)
Operator Inference for Non-Intrusive Model Reduction of Systems with Non-Polynomial Nonlinear Terms. Computer Methods in Applied Mechanics and Engineering
143, 104741, 9 pages (2020)
Identification of Port-Hamiltonian Systems from Frequency Response Data. Systems & Control Letters