GAMM Juniors Summer School on Applied Mathematics and Mechanics at the Max Planck Institute Magdeburg
Learning Models from Data: 75 Junior Scientists from 17 countries are virtually discussing on Model Reduction, System Identification and Machine Learning
The 7th Summer School on Applied Mathematics and Mechanics will be held from 27 to 31 July 2020 at the Max Planck Institute for Dynamics of Complex Technical Systems in Magdeburg. It will focus on Model Reduction, System Identification and Machine Learning. The event has been organized by the GAMM Juniors, these are young researchers and members of the International Association of Applied Mathematics and Mechanics (GAMM). The meeting will be held virtually because of the restrictions due to the COVID-19 Pandemic. 75 participants from 17 countries could therefore register for the online conference.
Developing models from data is a crucial part in many disciplines like engineering, medical research or social sciences. One of the major challenges consists in deriving a model which accurately describes a real-world phenomenon. However, in many applications a purely physics-based model may not be available or would be too complex to derive. For this reason, developing and investigating methods with which models can learn through data is of particular interest.
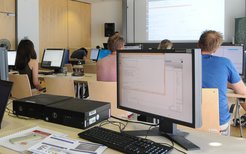
In order to reduce large-scale data to its most relevant information, techniques from model reduction can be utilized. Depending on the problem setting and the application, there exists a large variety of model reduction techniques such as proper orthogonal decomposition, reduced basis methods, rational interpolation, and balanced truncation, for instance. Most of them are projection-based methods identifying lowdimensional structures which are dominant for the process dynamics. Furthermore, in data-driven model reduction, the goal is to find reduced-order models which approximate the data. Some of the methods are the Loewner framework, vector fitting, Koopman operator approximation techniques, dynamic mode decomposition, operator inference, and time-lagged independent component analysis. From the field of systems and control theory, system identification methods (e.g., eigensystem realization algorithm) were derived in order to find exact models reproducing the measured input-output behavior of a system, without having access to the system's matrices.
The aim of this summer school is to study recent developments in the area of learning models from data, which will be presented by three top-level experts in online lectures and virtual exercise classes. Furthermore, the participants can present their own research in three virtual poster sessions.
Text: Carmen Gräßle / Petar Mlinarić / Yannik Heine