MPI Colloquia Series: Prof. Yoshiaki Kawajiri, Nagoya University: Uncertainty quantification for adsorption and chromatographic process models
Prof. Yoshiaki Kawajiri, Nagoya University
- Date: Jan 11, 2024
- Time: 02:00 PM - 04:00 PM (Local Time Germany)
- Speaker: Prof. Yoshiaki Kawajiri, Process Systems Engineering, Nagoya University, Japan
- Location: Max-Planck-Institut Magdeburg
- Room: Big Seminar Room "Prigogine"
- Host: MPI Scientific Coordination
- Contact: oelbermann@mpi-magdeburg.mpg.de
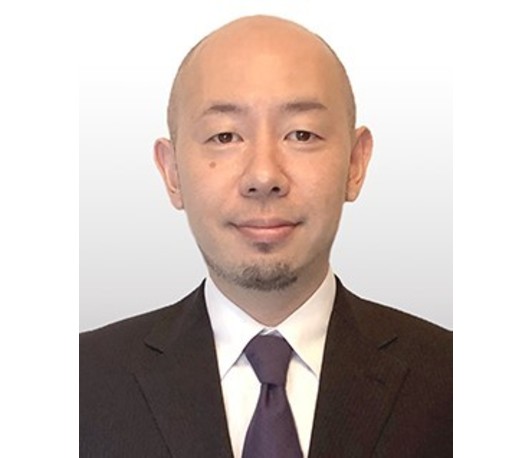
The Max Planck Institute Magdeburg invites you to its series of colloquia.
Top-class scientists, from notable German and worldwide research
institutions, give a survey of their research work.
Everybody who is interested, is invited to attend.
Dial-in via Zoom: https://eu02web.zoom-x.de/j/7302508065
Abstract
Developing a chemical process requires a robust mathematical model to predict the performance accurately. To increase the robustness, data should be obtained carefully so that parameters in the models can be obtained efficiently and accurately. Numerous challenges arise in modeling complex chemical processes. Adsorption and chromatographic processes, for instance, are intrinsically dynamic processes that do not have a steady state, where complex equilibrium as well as kinetics must be modeled carefully so that sufficiently accurate predictions can be given. Many experimental tests must be carried out to obtain data that reveal the equilibrium and mass transfer mechanisms.
To facilitate robust process design and operation, uncertainties in mathematical models should be quantified. However, uncertainty quantification can be challenging for many chemical processes. Model uncertainties are often ignored or quantified approximately by linearizing the model. Such approaches may not ensure sufficient robustness.
In this presentation, Bayesian estimation techniques are applied to estimate model parameters and quantify model uncertainties. By applying the Bayesian principle, model parameters are estimated as posterior probability distributions, utilizing prior knowledge as prior probability distributions. This quantification can be realized by a sequential Monte Carlo sampling technique that reduces computational effort. Some case studies are presented including adsorption isotherms and chromatographic processes. The flexibility of this framework is demonstrated in handling multiple data sources effectively in a hierarchical manner, while quantifying differences among data sets.
About the speaker (website)
Professor Kawajiri, an outstanding expert in the field of materials process technology. He is Professor of Materials Process Engineering and Professor at Research Center for Net Zero Carbon Society (joint appointment) at Nagoya University. With degrees from Kansai University (Japan) and a Ph.D. from Carnegie Mellon University (USA), Professor Kawajiri has spent his professional career at various prestigious institutions, including as a postdoctoral fellow at the Max Planck Institute itself (2007-2008). His extensive experience includes positions as R&D engineer at Organo Corporation in Japan, assistant and associated (tenured) professor at Georgia Institute of Technology, and most recently as visiting professor at Lappeenranta-Lahti University of Technology in Finland.
Professor Kawajiri's research focuses on the development of mathematically robust models to accurately predict the performance of chemical processes, with a particular focus on separation and purification processes such as adsorption and chromatography. He has received considerable recognition in the scientific community, including the Alexander von Humboldt Research Fellowship and several prestigious awards. He is also active in various scientific bodies, including as editor of 'Chemical Engineering Science' and board member of the International Adsorption Society.